Dual Contradistinctive Generative Autoencoder
Gaurav Parmar Dacheng Li Kwonjoon Lee Zhuowen Tu
Carnegie Mellon University UC San Diego
[Paper] | [GitHub]
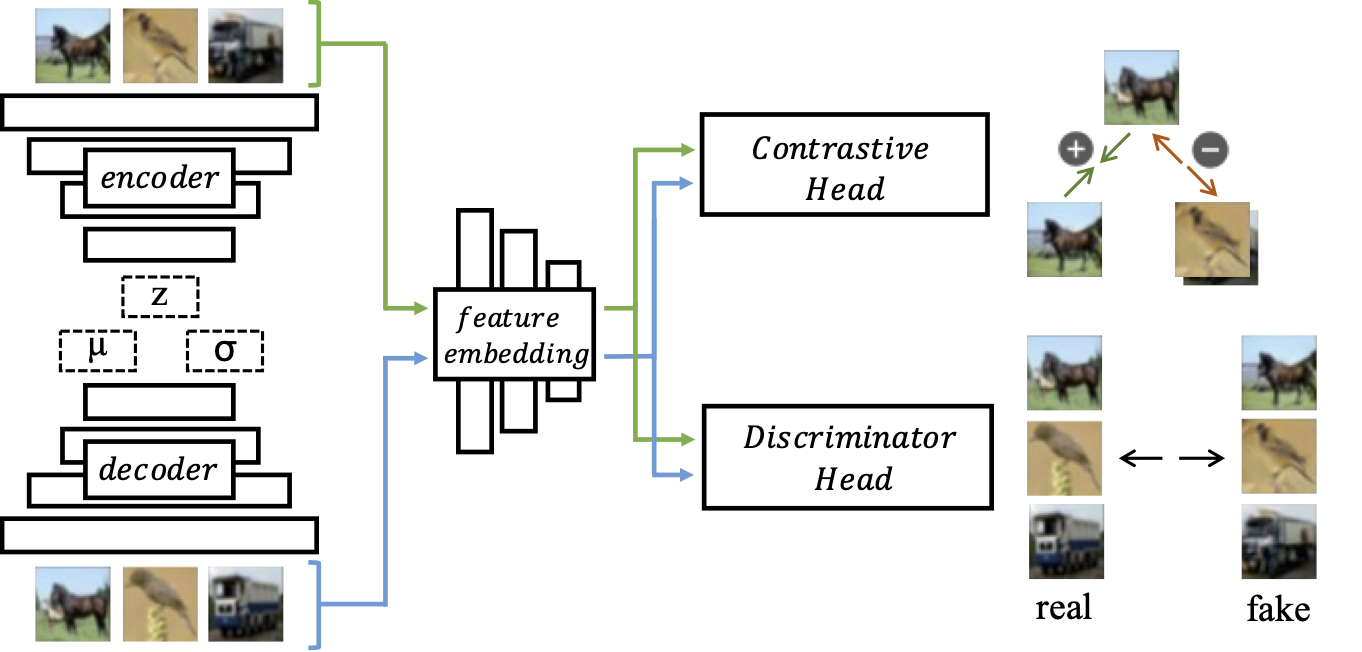
Abstract
We present a new generative autoencoder model with dualcontradistinctive losses to improve generative autoencoder that performs simultaneous inference (reconstruction) and synthesis (sampling). Our model, named dual contradistinctive generative autoencoder (DC-VAE), integrates an instance-level discriminative loss (maintaining the instance-level fidelity for the reconstruction/synthesis) with a set-level adversarial loss (encouraging the set-level fidelity for the reconstruction/synthesis), both being contradistinctive. Extensive experimental results by DC-VAE across different res-olutions including 32 x 32, 64 x 64, 128 x 128, and 512 x 512 are reported. The two contradistinctive losses in VAE work harmoniously in DC-VAE leading to a significant qualitative and quantitative performance enhancement over the baseline VAEs without architectural changes. State-of-the-art or competitive results among generative autoencoders for image reconstruction, image synthesis, image interpolation,and representation learning are observed. DC-VAE is ageneral-purpose VAE model, applicable to a wide variety of downstream tasks in computer vision and machine learning.
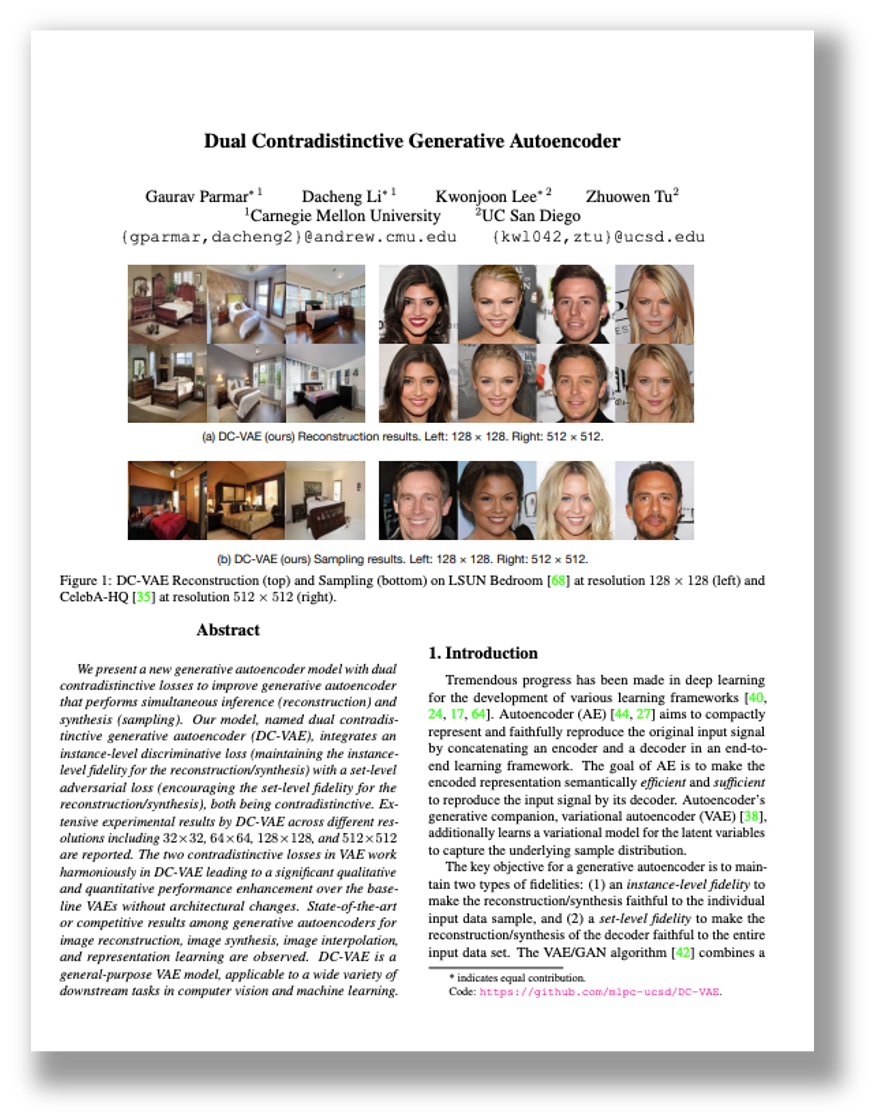
Paper
arXiv 2011.10063, 2021.
Citation
Gaurav Parmar, Dacheng Li, Kwonjoon Lee and Zhuowen Tu. "Dual Contradistinctive Generative Autoencoder", in CVPR, 2021.
Bibtex
DC-VAE Reconstruction and Sampling Results on LSUN Bedrooms and CelebA-HQ
![]() |
Acknowledgment
This work is funded by NSF IIS- 1717431 and NSF IIS-1618477. We thank Qualcomm Inc. for an award support. The work was performed when G. Parmar and D. Li were with UC San Diego.